Support Vector Machines#
We wil implement both hard-margin SVMs and soft-margin SVMs from scratch on a toy dataset. Apart from NumPy
, we would need to take the help of SciPy
for solving the quadratic programming problem.
Hard-Margin SVM#
import numpy as np
import matplotlib.pyplot as plt
plt.rcParams['figure.figsize'] = [6, 6]
#### DATA: DO NOT EDIT THIS CELL ####
X = np.array([[1, -3], [1, 0], [4, 1], [3, 7], [0, -2],
[-1, -6], [2, 5], [1, 2], [0, -1], [-1, -4],
[0, 7], [1, 5], [-4, 4], [2, 9], [-2, 2],
[-2, 0], [-3, -2], [-2, -4], [3, 10], [-3, -8]]).T
y = np.array([1, 1, 1, 1, 1,
1, 1, 1, 1, 1,
-1, -1, -1, -1, -1,
-1, -1, -1, -1, -1])
Understand the data#
\(\mathbf{X}\) is a data-matrix of shape \((d, n)\). \(\mathbf{y}\) is a vector of labels of size \((n, )\). Specifically, let us look at the shapes of the arrays involved.
d, n = X.shape
d, n
(2, 20)
y
array([ 1, 1, 1, 1, 1, 1, 1, 1, 1, 1, -1, -1, -1, -1, -1, -1, -1,
-1, -1, -1])
Visualize the dataset#
Let us now visualize the dataset given to us using a scatter plot. We will colour points which belong to class \(+1\) \(\color{green}{\text{green}}\) and those that belong to \(-1\) \(\color{red}{\text{red}}\). Following this, we shall inspect the data visually and determine its linear separability.
y_color = np.where(y == 1, 'green', 'red')
print(y)
print(y_color)
[ 1 1 1 1 1 1 1 1 1 1 -1 -1 -1 -1 -1 -1 -1 -1 -1 -1]
['green' 'green' 'green' 'green' 'green' 'green' 'green' 'green' 'green'
'green' 'red' 'red' 'red' 'red' 'red' 'red' 'red' 'red' 'red' 'red']
plt.scatter(
X[0, :], X[1, :],
c = y_color
)
plt.axhline(
color = 'black',
linestyle = '--',
linewidth = 0.5
)
plt.axvline(
color = 'black',
linestyle = '--',
linewidth = 0.5
);
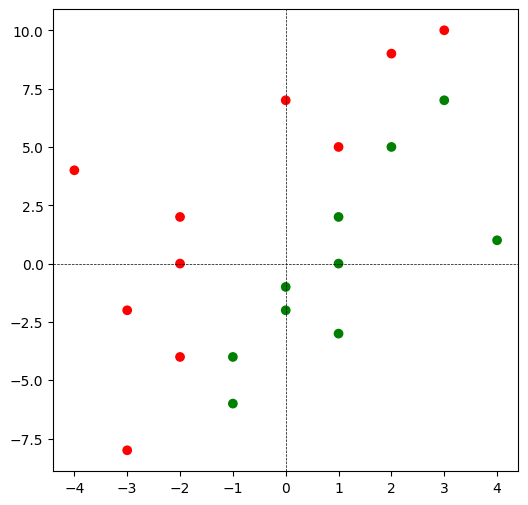
Linear Separability#
Is there another way to determine linear separability? Yes! We can train a perceptron and see if it converges in a finite amount of time. But a caveat: this method can be used to verify linear separability. It cannot be used to prove linear separability.
correct = 0
i = 0
w = np.zeros(d)
epochs = 0
while correct != n:
# prediction
y_hat = 1 if w @ X[:, i] >= 0 else -1
# mistake or not
if y[i] != y_hat:
w += X[:, i] * y[i]
correct = 0
else:
correct += 1
i += 1
# cycle back
if i == n:
i = 0
epochs += 1
print(f'converges in {epochs} epochs')
w /= np.linalg.norm(w)
w
converges in 4 epochs
array([ 0.94174191, -0.3363364 ])
Let us now visualize the perceptron’s weight vector and the corresponding decision boundary.
plt.scatter(
X[0, :],
X[1, :],
c = y_color
)
plt.axhline(
color = 'black',
linestyle = '--',
linewidth = 0.5)
plt.axvline(
color = 'black',
linestyle = '--',
linewidth = 0.5)
x_db = np.linspace(-4, 4)
y_db = -w[0] / w[1] * x_db
plt.plot(x_db, y_db, color = 'black')
plt.arrow(
0, 0, w[0], w[1],
head_width = 0.3,
head_length = 0.3)
plt.xlim(-6, 6)
plt.ylim(-6, 6);
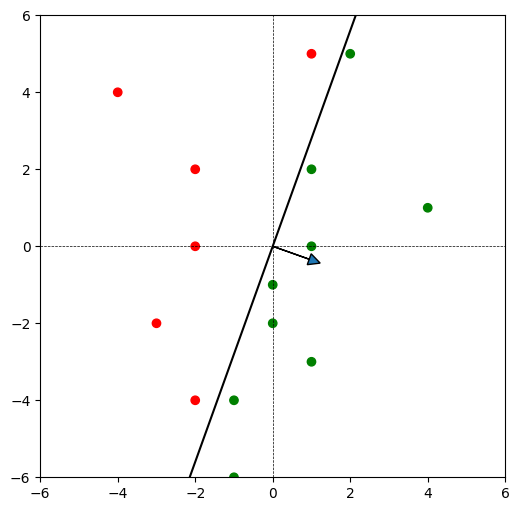
Computing the Dual Objective#
We shall follow a step-by-step approach to computing the dual objective function.
Step-1#
We compute the object \(\mathbf{Y}\) that appears in the dual problem.
Y = np.diag(y)
Y.shape
(20, 20)
Step-2#
Let \(\boldsymbol{\alpha}\) be the dual variable. The dual objective is of the form:
Next, we compute the matrix \(\mathbf{Q}\) for this problem.
Q = Y.T @ X.T @ X @ Y
Q.shape
(20, 20)
Step-3#
Since SciPy
’s optimization routines take the form of minimizing a function, we will recast \(f\) as follows:
We now have to solve :
Note that \(\max\) changes to \(\min\) since we changed the sign of the objective function.
def f(alpha):
return 0.5 * alpha @ Q @ alpha - alpha.sum()
Optimize#
Finally, we have most of the ingredients to solve the dual problem:
Find the optimal value, \(\boldsymbol{\alpha^{*}}\).
from scipy import optimize
alpha_init = np.zeros(n)
res = optimize.minimize(
f,
alpha_init,
bounds = optimize.Bounds(0, np.inf))
res
message: CONVERGENCE: REL_REDUCTION_OF_F_<=_FACTR*EPSMCH
success: True
status: 0
fun: -4.99999999958859
x: [ 0.000e+00 0.000e+00 ... 1.714e+00 1.629e+00]
nit: 30
jac: [ 5.000e+00 2.000e+00 ... 2.964e-04 -2.436e-04]
nfev: 735
njev: 35
hess_inv: <20x20 LbfgsInvHessProduct with dtype=float64>
alpha_star = res.x
alpha_star
array([0. , 0. , 0. , 0. , 0. ,
0. , 1.64285525, 1.65714065, 1.67142753, 1.68571355,
0. , 0. , 0. , 0. , 0. ,
0. , 0. , 0. , 1.71428422, 1.62856756])
Support vectors#
Let us find all the support vectors. Recall that support vectors are those points for which \(\alpha_i^{*} > 0\).
X_sup = X[:, alpha_star > 0]
y_sup = y[alpha_star > 0]
y_sup_color = np.where(y_sup == 1, 'green', 'red')
print(y_sup.shape[0])
6
We see that there are \(6\) support vectors.
Optimal weight vector (Primal solution)#
Let us now find the optimal weight vector \(\mathbf{w}^*\).
w_star = X @ Y @ alpha_star
w_star
array([ 2.99998762, -1.00002588])
This is pretty close to \((3, -1)\).
Decision Boundary#
Now we plot the decision boundary along with the supporting hyperplanes. Note where the support vectors lie in this plot.
def plot_db(w):
plt.scatter(
X[0, :],
X[1, :],
c = y_color, alpha = 0.3
)
plt.scatter(
X_sup[0, :],
X_sup[1, :],
c = y_sup_color
)
plt.axhline(
color = 'black',
linestyle = '--',
linewidth = 0.5
)
plt.axvline(
color = 'black',
linestyle = '--',
linewidth = 0.5
)
x_db = np.linspace(-4, 4)
y_db = -w[0] / w[1] * x_db
# decision boundary
plt.plot(
x_db, y_db,
color = 'black'
)
# supporting hyperplanes
y_sup_1 = 1 / w[1] - w[0] / w[1] * x_db
y_sup_2 = -1 / w[1] - w[0] / w[1] * x_db
plt.plot(
x_db, y_sup_1,
color = 'gray',
linestyle = '--'
)
plt.plot(
x_db, y_sup_2,
color = 'gray',
linestyle = '--'
)
plt.arrow(
0, 0, w[0], w[1],
head_width = 0.3,
head_length = 0.3
)
plt.xlim(-10, 10)
plt.ylim(-10, 10)
plot_db(w_star)
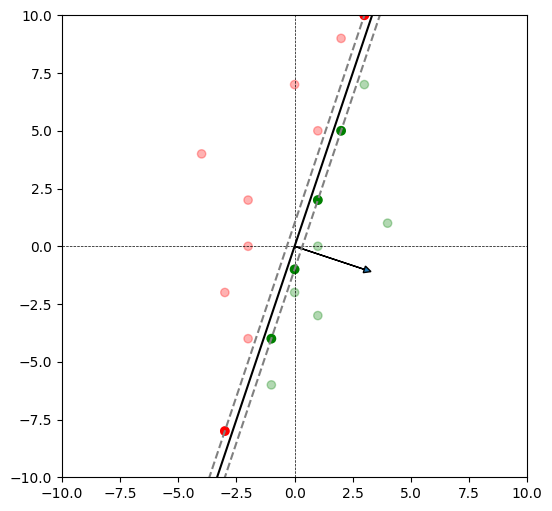
Note that the support vector appear darker than the rest of the points.
Soft-margin SVM#
We now turn to soft-margin SVMs. Adapt the hard-margin code that you have written for the soft-margin problem. The only change you have to make is to introduce an upper bound for \(\boldsymbol{\alpha}\), which is the hyperparameter \(C\).
#### DATA: DO NOT EDIT THIS CELL ####
X = np.array([[1, -3], [1, 0], [4, 1], [3, 7], [0, -2],
[-1, -6], [2, 5], [1, 2], [0, -1], [-1, -4],
[0, 7], [1, 5], [-4, 4], [2, 9], [-2, 2],
[-2, 0], [-3, -2], [-2, -4], [3, 10], [-3, -8],
[0, 0], [2, 7]]).T
y = np.array([1, 1, 1, 1, 1,
1, 1, 1, 1, 1,
-1, -1, -1, -1, -1,
-1, -1, -1, -1, -1,
1, 1])
d, n = X.shape
d, n
(2, 22)
Relationship between \(C\) and margin#
We now plot the decision boundary and the supporting hyperplane for the following values of \(C\).
(1) \(C = 0.01\)
(2) \(C = 0.1\)
(3) \(C = 1\)
(4) \(C = 10\)
We shall plot all of them in a \(2 \times 2\) subplot and study the tradeoff between the following quantities:
(1) Width of the margin.
(2) Number of points that lie within the margin or on the wrong side. This is often called margin violation.
# example of a lambda function
f = lambda x: x ** 2
f(10)
100
plt.rcParams['figure.figsize'] = [10, 10]
def train(C):
d, n = X.shape
# Step-1
Y = np.diag(y)
# Step-2
Q = Y.T @ X.T @ X @ Y
# Step-3
f = lambda alpha: 0.5 * alpha.T @ Q @ alpha - alpha.sum()
# Optimize
alpha_init = np.zeros(n)
res = optimize.minimize(
f,
alpha_init,
bounds = optimize.Bounds(0, C)
)
alpha_star = res.x
# Weight vector
w_star = X @ Y @ alpha_star
# Support vectors
X_sup = X[:, alpha_star > 0]
y_sup = y[alpha_star > 0]
y_sup_color = np.where(y_sup == 1, 'green', 'red')
return (alpha_star, w_star,
X_sup, y_sup, y_sup_color
)
def plot_db(w):
# Scatter plot for the dataset
y_color = np.where(y == 1, 'green', 'red')
plt.scatter(
X[0, :], X[1, :],
c = y_color, alpha = 0.3
)
plt.scatter(
X_sup[0, :], X_sup[1, :],
c = y_sup_color
)
plt.axhline(
color = 'black',
linestyle = '--',
linewidth = 0.5
)
plt.axvline(
color = 'black',
linestyle = '--',
linewidth = 0.5
)
# decision boundary
x_db = np.linspace(-4, 4)
y_db = -w[0] / w[1] * x_db
plt.plot(x_db, y_db, color = 'black')
# supporting hyperplanes
y_sup_1 = 1 / w[1] - w[0] / w[1] * x_db
y_sup_2 = -1 / w[1] - w[0] / w[1] * x_db
plt.plot(
x_db, y_sup_1,
color = 'gray',
linestyle = '--'
)
plt.plot(
x_db, y_sup_2,
color = 'gray',
linestyle = '--'
)
plt.title(f'C = {C}')
plt.xlim(-10, 10)
plt.ylim(-10, 10)
count = 1
for C in [0.01, 0.1, 1, 10]:
alpha_star, w_star, X_sup, y_sup, y_sup_color = train(C)
plt.subplot(2, 2, count)
plot_db(w_star)
count += 1
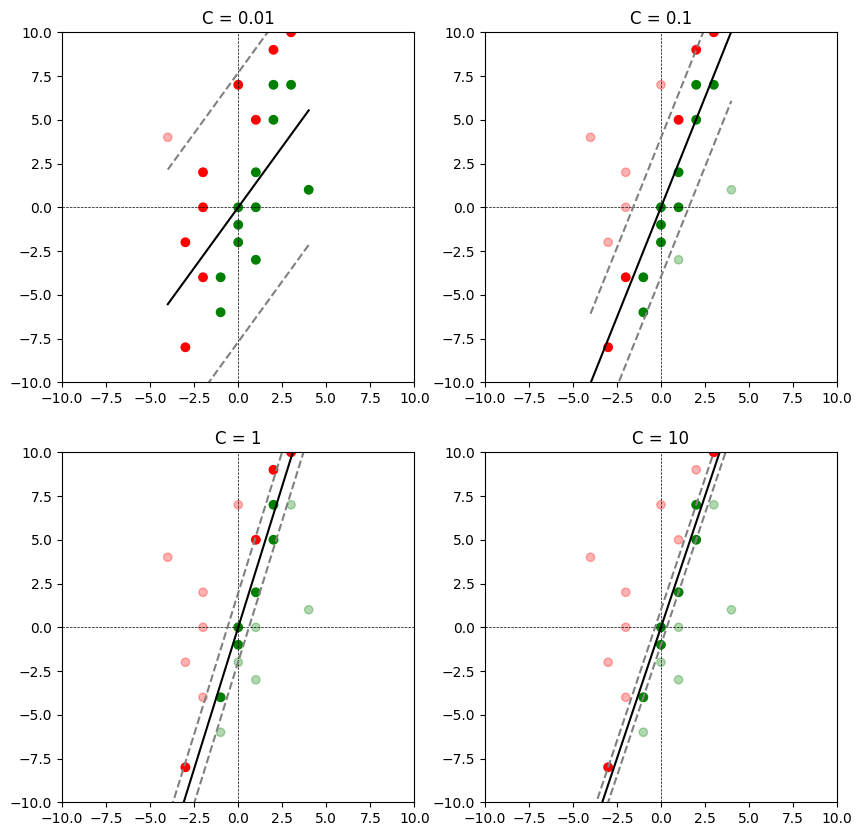
As \(C\) increases, the width of the margin decreases and fewer points violate the margin. Ideally, we would have to finetune for \(C\) using a validation dataset or using cross validation.