OPPE | T2-2024 | Solutions#
Instructions#
There are \(17\) NAT questions with marks distributed as \(1 \times 2 + 16 \times 3 = 50\).
Answers to all questions are going to be integers.
Solve the problem in the colab and enter the answer in the portal.
Always run the data-cell before running the solution cell.
In questions where a data-matrix \(\mathbf{X}\) and label-vector \(\mathbf{y}\) are mentioned, the data-cell will have the corresponding entries as
X
andy
.All other vectors, matrices and scalars necessary for solving the problem will be given in the data-cell. They will have the same name as the ones mentioned in the problem statement.
round
is a function that can be used to find the nearest integer. For example,round(1.2) == 1
andround(1.9) == 2
.
Notation#
The data-matrix in all problems will be of shape \(d \times n\), where \(d\) is the number of features and \(n\) is the number of data-points.
If \(\mathbf{x} = (1, 2, 3)\) is a vector, \(1, 2\) and \(3\) are termed the components of \(\mathbf{x}\). The sum of the components of \(\mathbf{x}\) is \(6\).
The norm of a vector \(\mathbf{x}\) is the Euclidean norm (\(L_2\)) by default. This is the only norm used in this exam.
All vectors will be represented as one-dimensional NumPy arrays. All matrices will be represented as two-dimensional NumPy arrays.
import numpy as np
import matplotlib.pyplot as plt
Question-1 [2 marks]#
\(\mathbf{X}\) is a data-matrix. If \(\boldsymbol{\mu}\) is the mean of the data-points, find the norm of \(\boldsymbol{\mu}\) and enter the nearest integer as your answer.
# DATA CELL
# DO NOT EDIT THIS
rng = np.random.default_rng(seed = 1001)
d = rng.integers(2, 10)
n = rng.integers(40, 50)
X = rng.integers(-5, 10, (d, n))
# Solution
# Run the data cell before running this
mu = X.mean(axis = 1)
round(np.linalg.norm(mu))
5
Question-2 [3 marks]#
\(\mathbf{X}\) is a data-matrix. Find the number of data-points whose norm is at least \(k\).
# DATA CELL
# DO NOT EDIT THIS
rng = np.random.default_rng(seed = 1002)
k = rng.integers(10, 20)
d = rng.integers(5, 10)
n = rng.integers(50, 100)
X = rng.integers(-10, 10, (d, n))
# Solution
# Run the data cell before running this
np.where(
np.linalg.norm(X, axis = 0) >= k,
1,
0
).sum()
17
Question-3 [3 marks]#
Consider a matrix \(\mathbf{A}\) of shape \(m \times n\). Find the trace of the matrix \(\mathbf{A}^T \mathbf{A}\), where the trace is the sum of the diagonal elements. Enter the nearest integer as the answer.
# DATA CELL
# DO NOT EDIT THIS
rng = np.random.default_rng(seed = 1003)
m, n = rng.integers(50, 100, 2)
A = rng.integers(-1, 2, (m, n))
# Solution
# Run the data cell before running this
M = A.T @ A
round(
sum(
[M[i][i] for i in range(M.shape[0])]
)
)
2517
Question-4 [3 marks]#
Consider the curves:
Find the number of points at which these two curves intersect in the interval \(0.15 \leqslant x \leqslant 0.5\).
# Solution
x = np.linspace(0.15, 0.5, 500)
plt.plot(x, x * np.exp(x))
plt.plot(x, np.sin(10 * np.pi * x))
print(4)
4
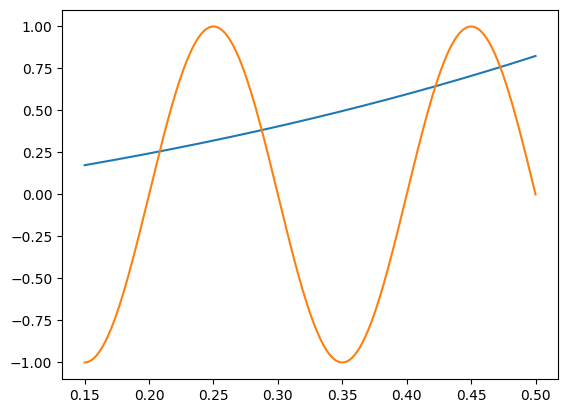
Question-5 [3 marks]#
Consider the system of equations \(\mathbf{Ax} = \mathbf{b}\), where:
If \(A\) is invertible, find the solution to this system, and enter the sum of the components of the solution as the answer. Your answer should be an integer.
# Solution
A = np.array([
[1, 2, -1, 3],
[0, 1, 2, -1],
[1, -2, 3, 1],
[0, -1, -1, 2]
])
b = np.array([1, 2, -1, 0])
assert np.linalg.matrix_rank(A) == A.shape[0]
x = np.linalg.inv(A) @ b
x.sum()
0.0
Question-6 [3 marks]#
Let \(\mathbf{w}\) be the weight vector of a linear classifier trained on a dataset for a binary classification problem with data-matrix \(\mathbf{X}\) and label vector \(\mathbf{y}\). The predicted label for a data-point \(\mathbf{x}\) defined as:
Find the predicted label vector and enter the sum of the components of the predicted label vector as the answer.
# DATA CELL
# DO NOT EDIT THIS
rng = np.random.default_rng(seed = 1006)
d = rng.integers(3, 6)
n = rng.integers(40, 60)
if n % 2 != 0: n += 1
X = rng.uniform(-2, 2, (d, n))
y = np.concatenate(
(np.ones(n // 2),
np.zeros(n // 2))
)
w = rng.uniform(-5, 5, d)
# Solution
# Run the data cell before running this
y_hat = np.where(w @ X >= 0, 1, 0)
y_hat.sum()
19
Common data for questions (7) to (10)#
Consider the following dataset for a binary classification problem. The data-matrix \(\mathbf{X}\) and the label vector \(\mathbf{y}\) are given below.
# DATA CELL
# DO NOT EDIT THIS
X = np.array([
[2, 2, 2, 3, -2, -2, -5, -3],
[0, 1, -1, 0, 0, -3, 3, -1]
])
y = np.array(
[-1, -1, -1, -1, 1, 1, 1, 1]
)
Question-7 [3 marks]#
Train a perceptron algorithm on this dataset. Cycle through the data-points from left to right, that is, \(i = 0\) to \(i = n - 1\). Make sure to strictly follow this cycle. If you make an update at data-point \(j\), proceed to data-point \(j + 1\) in the next iteration. Once you reach \(n - 1\), cycle back to \(0\).
Find the sum of the components of the weight vector and enter the nearest integer as your answer.
# Solution
# Run the data cell before running this
d, n = X.shape
i = cycle = 0
w = np.zeros(d)
while cycle != n:
y_hat = 1 if X[:, i] @ w >= 1 else -1
if y_hat != y[i]:
w += X[:, i] * y[i]
cycle = 0
else:
cycle += 1
i += 1
if i == n:
i = 0
w.sum()
-2.0
Question-8 [3 marks]#
In the context of hard-margin SVM, find the optimal \(\boldsymbol{\alpha}^{*}\) by solving the following dual optimization problem.
If the sum of the components of \(\boldsymbol{\alpha}^{*}\) is \(s\), find \(\cfrac{1}{s}\) and enter the nearest integer to \(\cfrac{1}{s}\) as the answer.
# Solution
# Run the data cell before running this
from scipy.optimize import minimize
from scipy.optimize import Bounds
Y = np.diag(y)
def f(alpha):
return (
0.5 * alpha @ Y.T @ X.T @ X @ Y @ alpha
- alpha.sum()
)
res = minimize(
f, x0 = np.zeros(n),
bounds = Bounds(0, np.inf)
)
alpha_star = res.x
round(1 / alpha_star.sum())
4
Question-9 [3 marks]#
Find the optimal weight vector \(\mathbf{w}^{*}\). If the sum of the components of \(\mathbf{w}^{*}\) is \(s\), find \(\cfrac{1}{s}\) and enter the nearest integer to \(\cfrac{1}{s}\) as the answer.
# Solution
w_star = X @ Y @ alpha_star
round(1 / w_star.sum())
-2
Question-10 [3 marks]#
Find the number of support vectors.
# Solution
(alpha_star > 0).sum()
5
Common data for questions (11) and (12)#
Consider a data-matrix \(\mathbf{X}\). Mean center it and perform linear PCA.
# DATA CELL
# DO NOT EDIT THIS
X = np.array([
[7, 5, -6, -11, 14.4, -2.8],
[10.5, 7.5, -9, -16.5, 21.6, -4.2],
[21, 15, -18, -33, 43.2, -8.4]
]).astype(np.float64)
# Solution
# Run the data cell before running this
d, n = X.shape
X -= X.mean(axis = 1).reshape(d, 1)
C = X @ X.T / n
eigval, eigvec = np.linalg.eigh(C)
var = np.flip(eigval)
pcs = np.flip(eigvec, axis = 1)
Question-11 [3 marks]#
If the first PC is \(\mathbf{w}_1\), let the sum of the components of \(\mathbf{w}_1\) be \(a\). Find the absolute value of \(7a\) and enter the nearest integer as your answer.
# Solution
round(np.abs(pcs[:, 0].sum() * 7))
11
Question-12 [3 marks]#
Find the variance along the first PC. Enter the nearest integer as your answer.
# Solution
round(var[0])
896
Question-13 [3 marks]#
Find the sum of the variances along the second and third PC. Enter the nearest integer as your answer.
# Solution
round(var[1:].sum())
0
Common data for questions (14) to (16)#
Consider a dataset \(\mathbf{X}\) for a clustering problem. Start with the initial means as \(\boldsymbol{\mu}_1 = (2, 3, 5)\) and \(\boldsymbol{\mu}_2 = (-3, -5, -7)\) and run K-means with \(k = 2\).
# DATA CELL
# DO NOT EDIT THIS
X = np.array([
[-8.2, 3.2, -5.1, 4., -7, 8.1, -2],
[-10.1, 4.1, -3.3, 2., -5, 6.2, -5],
[-3.4, 4.9, -3, 2., -5, 6.5, -5.3]
])
# Solution
mus = np.array([
[2., -3.],
[3., -5.],
[5., -7.]
])
d, n = X.shape
z_prev = np.ones(n)
z = np.zeros(n)
k = 2
while not np.array_equal(z_prev, z):
z_prev = z.copy()
for i in range(n):
dsq = (mus - X[:, i].reshape(d, 1)) ** 2
z[i] = np.argmin(dsq.sum(axis = 0))
for j in range(k):
if np.any(z == j):
mus[:, j] = X[:, z == j].mean(axis = 1)
mus
array([[ 5.1 , -5.575 ],
[ 4.1 , -5.85 ],
[ 4.46666667, -4.175 ]])
Question-14 [3 marks]#
Find the norm of the final mean \(\boldsymbol{\mu}_1\). Enter the nearest integer as your answer.
# Solution
round(np.linalg.norm(mus[:, 0]))
8
Question-15 [3 marks]#
Find the norm of the final mean \(\boldsymbol{\mu}_2\). Enter the nearest integer as your answer.
round(np.linalg.norm(mus[:, 1]))
9
Question-16 [3 marks]#
Find the cluster to which the data-point \((0, 1, 2)\) belongs. Enter \(1\) if it is closer to \(\boldsymbol{\mu}_1\) than \(\boldsymbol{\mu}_2\) and \(2\) otherwise.
x = np.array([0, 1, 2])
dsq = (mus - x.reshape(d, 1)) ** 2
np.argmin(dsq.sum(axis = 0)) + 1
1
Question-17 [3 marks]#
Fit a linear regression model on the dataset \((\mathbf{X}, \mathbf{y})\) and find the optimal weight vector \(\mathbf{w}^{*}\) using the normal equations. Enter the nearest integer to the sum of the components of \(\mathbf{w}^{*}\) as the answer.
# DATA CELL
# DO NOT EDIT THIS
X = np.array([
[1., -1., 3., -2., 1.],
[0., -2., 1., 0., 3.],
[-2., 1., 0., 1., 2.],
[1., -2., 3., -1., 4.]
])
y = np.array(
[3.1, 4.9, -2.5, 10.3, -4.2]
)
d, n = X.shape
w = np.linalg.pinv(X.T) @ y
round(w.sum())
-9